Assessments have long been a cornerstone of education, hiring, and psychological research. However, with advancements in artificial intelligence and machine learning, these assessments are evolving faster than ever. Moreover, Big Data is revolutionizing how we measure personality, cognitive ability, and behavioral traits. From adaptive testing to bias detection, it is improving accuracy, efficiency, and fairness in psychological measurement. But with these advancements come challenges—concerns about privacy, bias, and ethical AI implementation.
In this article, we’ll break down how Big Data is transforming in testing, where it’s being applied, and what the future holds.
What is Big Data?
Big Data refers to large-scale, complex datasets that can be analyzed to reveal patterns, trends, and associations. In psychometrics, this means massive amounts of test-taker data—not just scores, but response times, keystroke dynamics, facial expressions, and even biometric data.
Traditional assessments relied on small samples and fixed test items. Today, platforms collect millions of data points in real time, enabling AI-driven insights that were previously impossible. Machine learning algorithms can analyze these rich datasets to detect patterns in behavior, predict future performance, and enhance the precision of assessments.
This shift has led to more precise, adaptive, and fairer assessments—but also raises ethical and practical concerns, such as data privacy, bias in algorithms, and the need for transparency in decision-making.
How Big Data is Transforming Assessment
1. Smarter Assessment
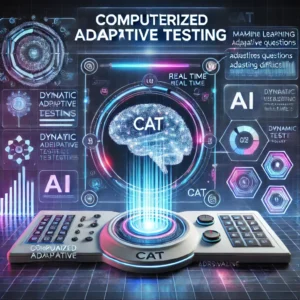
Big Data is used to drive the development, administration, and scoring of future-focused assessments. Some examples of this are process data, machine learning personalization, and adaptive testing. By leveraging data-driven decision making inside the assessment, we can make it smarter, faster, and fairer.
Process data refers to the use of data other than answer selection, such as keystroke/mouse dynamics. An example is a drag-and-drop question where a student has to classify animals into Reptile, Mammal, or Amphibian; instead of just recording the final locations, we can evaluate what they dragged first and where, if they changed their mind, how much time they took to answer the question, etc. This can provide greater insight into both scoring and feedback. We can also evaluate the use of tools like rulers or calculators (Liao & Sahin, 2020). However, it might be of limited use in high-stakes exams where the final answer is what matters.
Machine learning algorithms based on Big Data can improve assessment by personalizing the assessment or even the selection of assessment. Next-generation learning systems are designed to be adaptive from a very high level, understanding what students know and recommending the next modules, not unlike how your favorite video streaming service learns what shows you like or not and recommends new ones. This could also be done inside the assessment, transforming it from assessment of learning (AoL) to assessment for learning (AfL).
Big Data can enhance Computerized Adaptive Testing (CAT), where test difficulty adjusts in real time based on the test-taker’s responses. Instead of presenting a fixed set of questions, the algorithm selects each new question based on the test-taker’s previous answers, making the assessment more efficient and tailored to individual ability levels. This approach has been utilized for decades with large-scale exams, with well-known benefits like shorter tests, reduced anxiety, and increased engagement. The Graduate Management Admission Test (GMAT) transitioned to a computer-adaptive format in 1997. Similarly, the Graduate Record Examination (GRE) introduced a computer-adaptive format in 1993 and made it mandatory in 1997. You can read more about adaptive testing in action here.
2. AI-Driven Personality and Behavioral Analysis
Psychometric models like the Big Five and HEXACO are now enhanced by machine learning and natural language processing (NLP).
AI can analyze how people respond, not just what they answer—including text responses, speech patterns, and decision-making behaviors. Companies like HireVue analyze facial expressions and speech cadence to assess job candidates’ traits.
3. Detecting Bias and Improving Fairness
One of the biggest concerns in psychological testing is bias. Traditional tests have been criticized for favoring certain demographics over others.
With Big Data, AI can flag biased questions by analyzing how different groups respond.
Example: If women or minority groups consistently underperform on a test question despite having equal qualifications, the item may be unfair and flagged for review.
This helps ensure that assessments are more equitable and inclusive.
4. Predicting Job Performance and Talent Retention
Companies are increasingly using psychometric Big Data to predict:
- Which candidates will succeed in a role
- Who is at risk of burnout
- How leadership potential can be identified early
Example: Google uses Big Data psychometric analysis to refine its hiring process, ensuring long-term employee success and cultural fit. You can read a more in-depth look at their hiring process here.
5. Real-Time Fraud Detection in Assessments
Just like SIFT detects test fraud, Big Data helps identify cheating in online exams. AI can analyze eye movement, response times, and typing behavior to detect suspicious activity. The models to do so are trained on large data sets; for example, a proctoring provider might have millions of past exam data, with stored videos as well as human-confirmed flags of if they were cheating or not. Universities and companies now use AI-powered proctoring tools to prevent cheating in high-stakes tests.
The Challenges of Big Data in Assessment
The integration of Big Data into psychometrics offers significant advancements but also presents several challenges that must be carefully managed:
1. Privacy and Data Security Risks
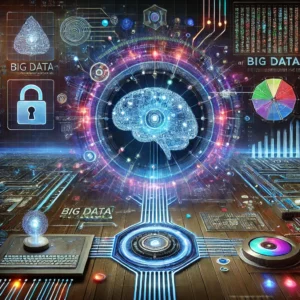
Collecting extensive psychological data introduces serious ethical concerns. Regulations like the General Data Protection Regulation (GDPR) in Europe and the Health Insurance Portability and Accountability Act (HIPAA) in the United States mandate strict data protection protocols to safeguard individuals’ personal information. Ensuring compliance with these laws is essential to protect test-takers’ confidentiality and maintain public trust.
2. Algorithmic Bias in AI
AI models trained on historical data can inadvertently perpetuate existing biases if not properly managed. A notable example is Amazon’s hiring algorithm, which was discontinued after it demonstrated bias against female candidates. This incident underscores the importance of developing strategies to identify and mitigate bias in AI-driven assessments to ensure fairness and equity. You can read more about the incident here.
3. Necessity of Human Oversight
While AI-driven assessments can provide valuable insights, human oversight remains crucial. Misinterpretation or overreliance on automated results can lead to incorrect hiring decisions, misdiagnoses, or unethical outcomes. Professionals in psychology and human resources play a vital role in interpreting data within the appropriate context, ensuring that decisions are informed by both technological tools and human judgment.
Addressing these challenges requires a balanced approach that leverages the benefits of Big Data and AI while implementing safeguards to protect individual rights and uphold ethical standards.
The Future of Big Data in Psychometrics
The integration of Big Data into psychometrics is paving the way for innovative advancements that promise to enhance the precision, security, and ethical standards of assessments. Here are some emerging trends:
1. Wearable Biometric Assessments
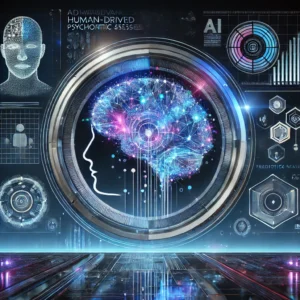
The incorporation of data from wearable devices, such as heart rate monitors and electroencephalogram (EEG) sensors, into cognitive tests is on the rise. These devices provide real-time physiological data that can offer deeper insights into a test-taker’s cognitive and emotional states, potentially leading to more comprehensive assessments. For instance, in healthcare, wearable technology has been utilized for continuous monitoring and personalized care, highlighting its potential application in psychometric evaluations.
2. More Transparent AI Models
The demand for explainable AI in assessments is growing. Developing AI models that provide clear, understandable explanations for their decisions is crucial to ensure ethical use and to mitigate biases. This transparency is essential for building trust in AI-driven assessments and for adhering to ethical standards in testing. You can learn about that here.
3. Blockchain for Secure Testing
Blockchain technology is being explored for enhancing the security and integrity of assessments. Its decentralized ledger system can be used for secure credential verification, ensuring that test results are tamper-proof and verifiable. This approach can uphold the integrity of assessments and protect against fraudulent activities.
4. Integration of Assessment with Learning
The future of testing includes a closer integration of assessment and learning processes. Adaptive learning platforms that utilize Big Data can provide personalized learning experiences, adjusting content and assessments in real-time to meet individual learner needs. This approach not only enhances learning outcomes but also provides continuous assessment data that can inform educational strategies.
5. Greater Insight into Open-Response Items
Advancements in natural language processing (NLP) and machine learning are enabling more sophisticated analysis of open-response items in assessments. These technologies can evaluate the content, structure, and sentiment of written responses, providing deeper insights into test-takers’ understanding, reasoning, and communication skills. This development allows for a more nuanced evaluation of competencies that are difficult to measure with traditional multiple-choice questions.
As Big Data continues to shape the field of psychometrics, it is imperative to balance innovation with ethical responsibility. Ensuring data privacy, mitigating biases, and maintaining transparency are crucial considerations as we advance toward more sophisticated and personalized assessment methodologies.
Final Thoughts: The Future is Here, but Caution is Key
Big Data is revolutionizing assessment, making tests faster, more precise, and highly adaptive. AI-driven testing can unlock deeper insights into human cognition and behavior, transforming everything from education to hiring. However, these advancements come with profound ethical dilemmas—issues of privacy, bias, and opaque AI decision-making must be addressed to ensure responsible implementation.
To move forward, assessment professionals must embrace AI’s potential while demanding fairness, transparency, and rigorous data governance. The future of testing is not just about innovation but about striking the right balance between technology and ethical responsibility. As we harness AI to enhance assessments, we must remain vigilant in protecting individuals’ rights and ensuring that data-driven insights serve to empower rather than exclude.