Confidence intervals (CIs) are a fundamental concept in statistics, used extensively in assessment and measurement to estimate the reliability and precision of data. Whether in scientific research, business analytics, or health studies, confidence intervals provide a range of values that likely contain the true value of a parameter, giving us a better understanding of uncertainty. This article dives into the concept of confidence intervals, how they are used in assessments, and real-world applications to illustrate their importance.
What Is a Confidence Interval?
CI is a range of values, derived from sample data, that is likely to contain the true population parameter. Instead of providing a single estimate (like a mean or proportion), it gives a range of plausible values, often expressed with a specific level of confidence, such as 95% or 99%. For example, if a survey estimates the average height of adult males to be 175 cm with a 95% CI of 170 cm to 180 cm, it means that we can be 95% confident that the true average height of all adult males falls within this range.
These are made by taking plus or minus a standard error times a factor, around the single estimate, creating a lower bound and upper bound for a range.
Upper Bound = Estimate + factor * standard_error
Lower Bound = Estimate – factor * standard_error
The value of the factor depends upon your assumptions and the desired percentage in the range. You might want 90%, 95%, 0r 99%. With the standard normal distribution, the factor is 1.96 (see any table of z-scores), which makes for easy quick math of 2 in your head to get a rough idea. So, in the example above, we might find that the average height for a sample of 100 adult males is 175 cm with a standard deviation of 25. The standard error of the mean is SD*sqrt(N), which in this case is 25*sqrt(100) = 2.5. If we take plus or minus 2 times the standard error of 2.5, that is how we get a confidence interval that the true population mean is 95% likely to be between 170 and 180.
This example is from general statistics, but confidence intervals are used for specific reasons in assessment.
How Confidence Intervals Are Used in Assessment and Measurement
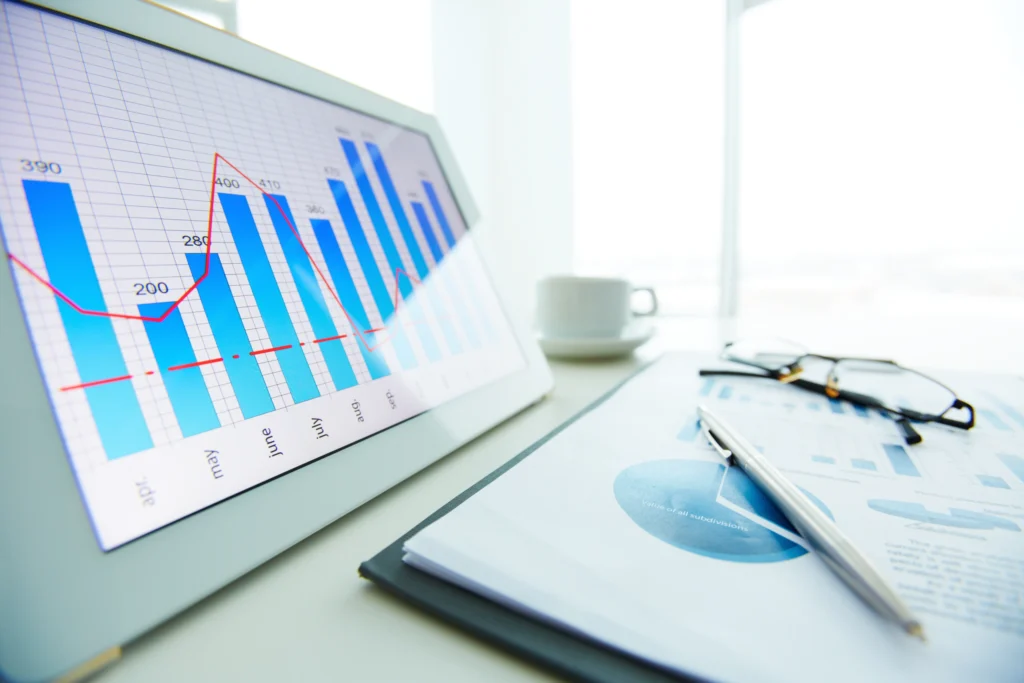
1. Statistical Inference
CIs play a crucial role in making inferences about a population based on sample data. For instance, researchers studying the effect of a new drug might calculate a CI for the average improvement in symptoms. This helps determine if the drug has a significant effect compared to a placebo.
2. Quality Control
In industries like manufacturing, CIs help assess product consistency and quality. For example, a factory producing light bulbs may use CIs to estimate the average lifespan of their products. This ensures that most bulbs meet performance standards.
3. Education and Testing
In educational assessments, CIs can provide insights into test reliability and student performance. For instance, a standardized test score might come with a CI to account for variability in test conditions or scoring methods.
Real-World Examples of Confidence Intervals
1. Medical Research
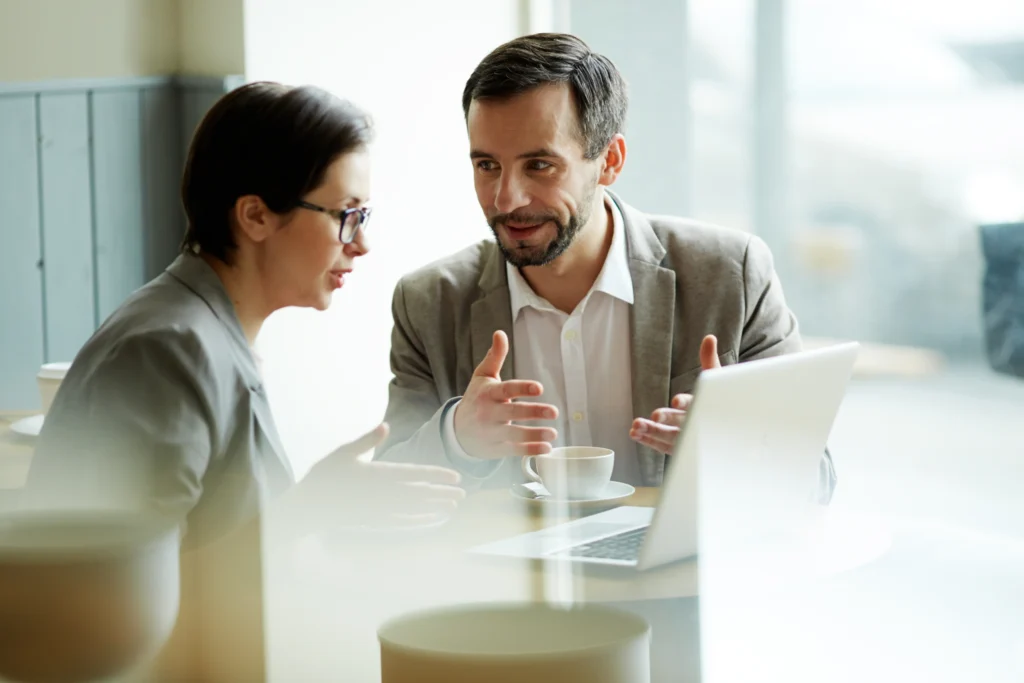
In clinical trials, CIs are often used to estimate the effectiveness of treatments. Suppose a study finds that a new vaccine reduces the risk of a disease by 40%, with a 95% CI of 30% to 50%. This means there’s a high probability that the true effectiveness lies within this range, helping policymakers make informed decisions.
2. Business Analytics
Businesses use CIs to forecast sales, customer satisfaction, or market trends. For example, a company surveying customer satisfaction might report an average satisfaction score of 8 out of 10, with a 95% CI of 7.5 to 8.5. This helps managers gauge customer sentiment while accounting for survey variability.
3. Environmental Studies
Environmental scientists use CIs to measure pollution levels or climate changes. For instance, if data shows that the average global temperature has increased by 1.2°C over the past century, with a CI of 0.9°C to 1.5°C, this range provides a clearer picture of the uncertainty in the estimate.
Confidence Intervals in Education: A Closer Look
CIs are particularly valuable in education, where they help assess the reliability and validity of test scores and other measurements. By understanding and applying CIs, educators, test developers, and policymakers can make more informed decisions that impact students and learning outcomes.
1. Estimating a Range for True Score
CIs are often paired with standard error of measurement (SEM) to provide insights into the reliability of test scores. SEM quantifies the amount of error expected in a score due to various factors like testing conditions or measurement tools. It gives us a range for a true score around the observed score (see technical note near then end on this).
For example, consider a standardized test with a scaled score range of 200 to 800. If a student scores 700 with an SEM of 20, the 95% CI for their true score is calculated as:
Score ± (SEM × Z-value for 95% confidence)
700 ± (20 × 1.96) = 700 ± 39.2700 ± (20 × 1.96) = 700 ± 39.2
Thus, the 95% CI is approximately 660 to 740. This means we can be 95% confident that the student’s true score lies within this range, accounting for potential measurement error. Because this is important, it is sometimes factored into important decisions such as setting a cutscore to be hired at a company based on a screening test.
The reasoning for this is accurately described by this quote from Prof. Michael Rodriguez, noted by Mohammed Abulela on LinkedIn:
A test score is a snapshot estimate, based on a sample of knowledge, skills, or dispositions, with a standard error of measurement reflecting the uncertainty in that score-because it is a sample. Fair test score interpretation employs that standard error and does not treat a score as an absolute or precise indicator of performance.
2. Using Standard Error of Estimate (SEE) for Predictions
The standard error of the estimate (SEE) is used to evaluate the accuracy of predictions in models, such as predicting student performance based on prior data.
For instance, suppose that a college readiness score ranges from 0 to 500, and is predicted by a student’s school grades and admissions test score. If a predictive model estimates a student’s college readiness score to be 450, with an SEE of 25, the 95% confidence interval for this predicted score is:
450 ± (25 × 1.96) = 450 ± 49
This results in a confidence interval of 401 to 499, indicating that the true readiness score is likely within this range. Such information helps educators evaluate predictive assessments and develop better intervention strategies.
3. Evaluating Group Performance
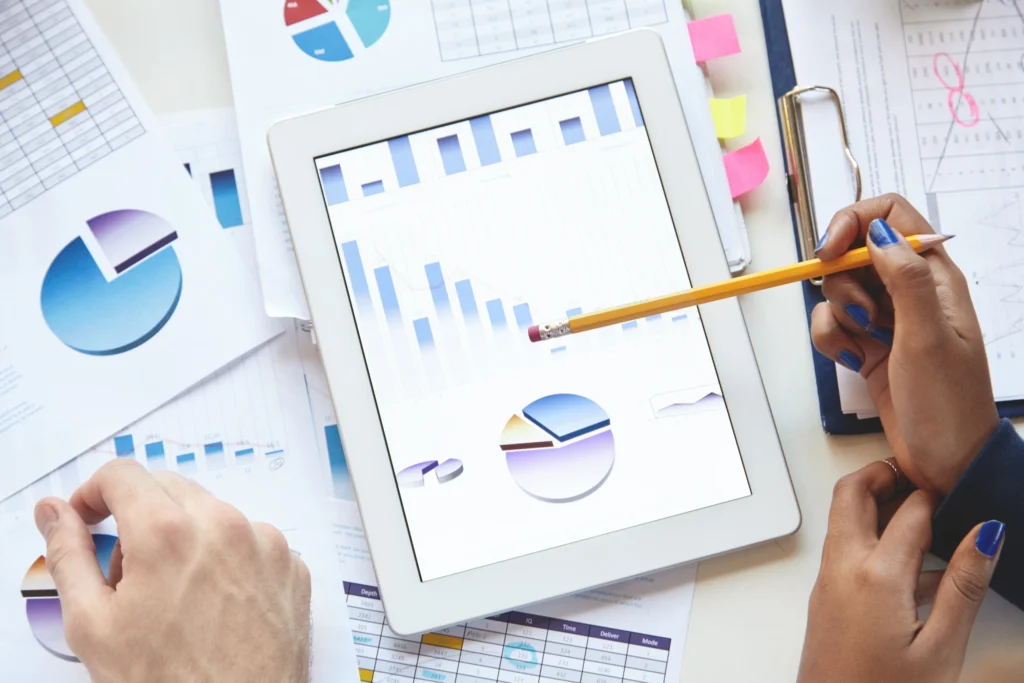
CIs are also used to assess the performance of groups, such as schools or districts. For instance, if a district’s average math score is 75 with a 95% CI of 73 to 77, policymakers can be fairly confident that the district’s true average falls within this range. This insight is crucial for making fair comparisons between schools or identifying areas that need improvement.
4. Identifying Achievement Gaps
When studying educational equity, CIs help measure differences in achievement between groups, such as socioeconomic or demographic categories. For example, if one group scores an average of 78 with a CI of 76 to 80 and another scores 72 with a CI of 70 to 74, the overlap (or lack thereof) in intervals can indicate whether the gap is statistically significant or might be due to random variability.
5. Informing Curriculum Development
CIs can guide decisions about curriculum and instructional methods. For instance, when pilot-testing a new teaching method, researchers might use CIs to evaluate its effectiveness. If students taught with the new method have scores averaging 85 with a CI of 83 to 87, compared to 80 (78 to 82) for traditional methods, educators might confidently adopt the new approach.
6. Supporting Student Growth Tracking
In long-term assessments, CIs help track student growth by providing a range around estimated progress. If a student’s reading level improves from 60 (58–62) to 68 (66–70), educators can confidently assert growth while acknowledging measurement variability.
Key Benefits of Using Confidence Intervals
- Enhanced Decision-Making: CIs provide a range, rather than a single estimate, making decisions more robust and informed.
- Clarity in Uncertainty: By quantifying uncertainty, confidence intervals allow stakeholders to understand the limitations of the data.
- Improved Communication: Reporting findings with CIs ensures transparency and builds trust in the results.
How to Interpret Confidence Intervals
A common misconception is that a 95% CI means there’s a 95% chance the true value falls within the interval. Instead, it means that if we repeated the study many times, 95% of the calculated intervals would contain the true parameter. Thus, it’s a statement about the method, not the specific interval. This is similar to the common misinterpretation of an experimental p-value that it is the probability that our alternative hypothesis is true; instead, it is the probability of our experiment’s results if the null is true.
Final Thoughts
CIs are indispensable in assessment and measurement, offering a clearer understanding of data variability and precision. By applying them effectively, researchers, businesses, and policymakers can make better decisions based on statistically sound insights.
Whether estimating population parameters or evaluating the reliability of a new method, CIs provide the tools to navigate uncertainty with confidence. Start using CIs today to bring clarity and precision to your analyses!