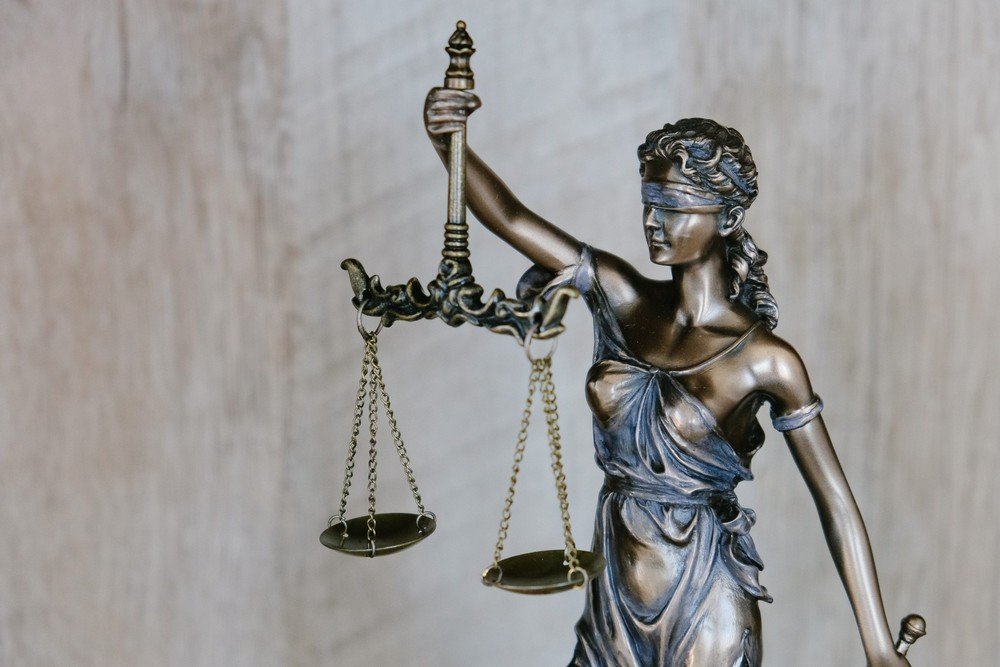
What Makes a Test Biased or Unfair?
Content
One of the primary goals of psychometrics and assessment research is to ensure that tests, their scores, and interpretations of the scores, are reliable, valid, and fair. The concepts of reliability and validity are discussed quite often and are well-defined, but what do we mean when we say that a test is fair or unfair? We’ll discuss it here. Though note that fairness is technically part of validity, because if there is bias, then the interpretations being made from scores are usually biased as well.
What do we mean by bias?
Well, there are actually three types of bias in assessment.
1. Differential item functioning (DIF)/ differential test functioning (DTF)
This type of bias occurs when a single item, or sometimes a test, is biased against a group when ability/trait level is constant. For example, suppose that the reference group (usually the majority) and focal group (usually a minority) perform similarly on the test overall, but on one item we find that the focal group was less likely to get the item correct after adjusting for total score performance. This is known as differential item functioning (DIF). Content experts should review the question.
2. Overall test bias
With this type of bias, we find that the entire test is biased against the focal group, so that they receive lower scores (ability/trait estimate) than the reference group. This is especially concerning if there is data from another test or variable that shows the two groups should be of equal ability. However, there are many cases where the focal group has lower scores not because the test is biased, but because of some other reason. For example, if it is economically disadvantaged and receives subpar educational opportunities, the test could very well be valid and simply reflect these well-known inequities.
3. Predictive bias
This is a complex situation. Suppose that the test itself was not biased, but it is used to predict something like job performance or university admissions, and the test scores systematically underpredict performance for the focal group. This is manifested in the predictive model, such as a linear regression, and not in the test scores. There is also selection bias, where a focal group ends up not being selected as often. In the USA, a rule of thumb is the four-fifths rule.
Other types of unfairness
There are other ways that a test can be considered unfair. One is the case of unequal precision. This refers to the situation that is the case with almost all traditional exams that there are plenty of items of middle difficulty, but not as many items that are easy or difficult. This can lead to very inaccurate scores for examinees on the top or bottom of the distribution. It is one reason that scaled scores are often capped on the ends of the spectrum; the difference between a person at the 98th percentile vs 99th percentile is most likely not meaningful, even if there is a wide difference in the raw scores.
Another is the case of test adaptation and translation. Here, the original test and its items might be unbiased, but when the test is translated or adapted to a different language or culture, it becomes biased. In such cases, the data might manifest itself as DIF/DTF or test bias as described above. I recall a story that a friend of mine told me about an item that was translated to Spanish, where the original item in English was quite strong and unbiased, but when used in Latin America it touched on a cultural aspect that was not present in USA/Canada, and performed poorly.
How can we find test bias?
Psychometricians have a number of statistical methods that are designed to specifically look for the situations described here. Differential item functioning in particular has a ton of scientific literature devoted to it. One example method, which is older but still commonly used, is the Mantel-Haenszel statistic. For predictive bias, I remember learning about the partial F-test in graduate school, but have not had the opportunity to perform such analyses since then.
How do we address or avoid test bias?
As with many things, an ounce of prevention is worth a pound of cure. High-stakes exams such as university admissions will invest heavily in avoiding bias. They will create detailed item writing guidelines, heavily train the item writers, and pay for items to be reviewed not only by experts but by people who are representative of target populations. Of course, some issues will always slip through this process, which is why it is important to perform the statistical analyses afterwards to validate the items, the test, and predictive models.
Where can I learn more?
Here are some relevant resources to help you learn more about test bias.
Handbook of Methods for Detecting Test Bias
Test Bias in Employment Selection Testing: A Visual Introduction
Nathan Thompson, PhD
Latest posts by Nathan Thompson, PhD (see all)
- What is a rubric? - February 13, 2025
- Time Limits in Assessment - February 8, 2025
- SIFT: A new tool for detection of test fraud - February 4, 2025