Education
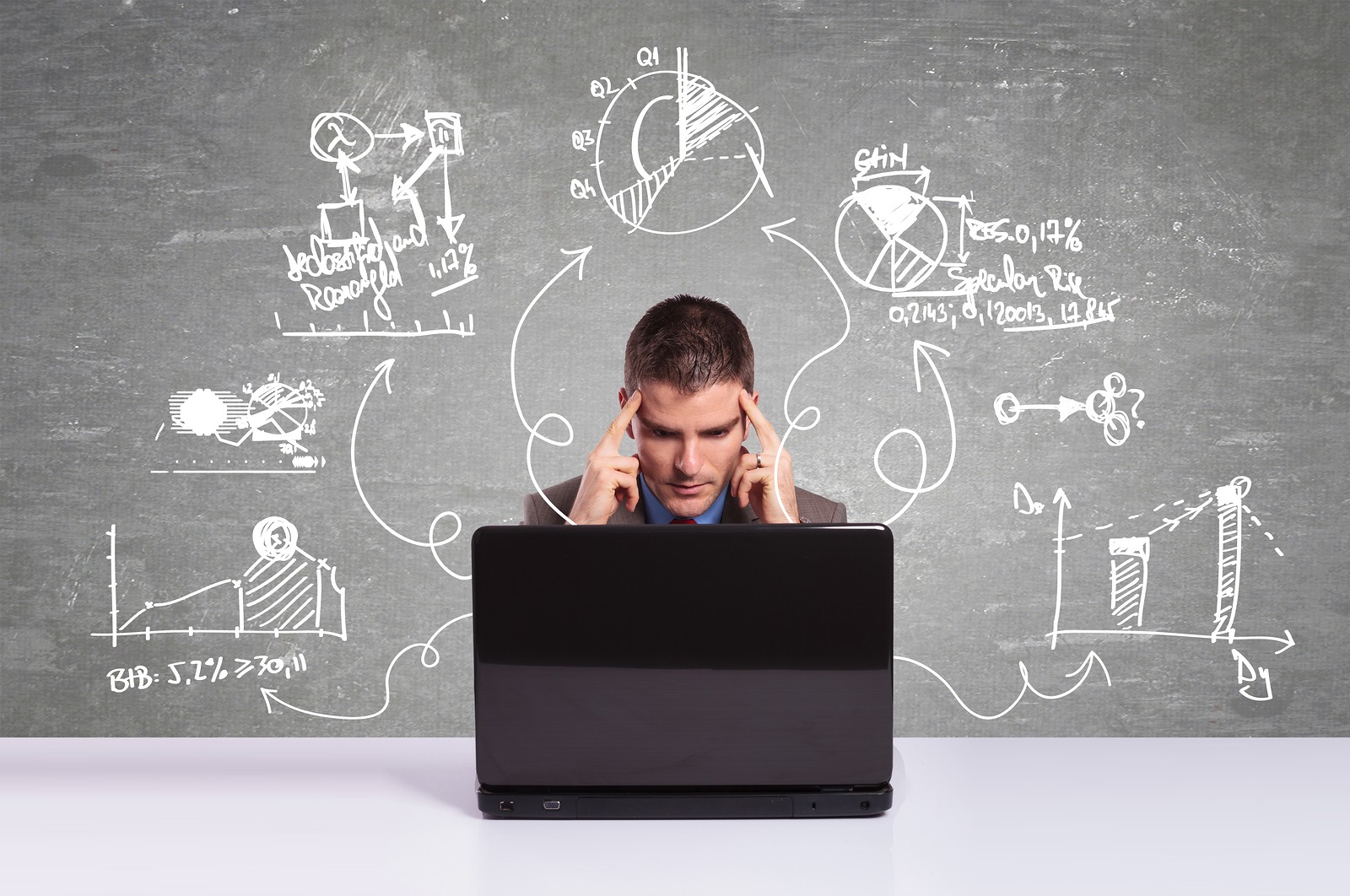
Automated Item Generation
Automated item generation (AIG) is a paradigm for developing assessment items (test questions), utilizing principles of artificial intelligence and automation. As the name suggests, it tries to automate some or all of the effort involved
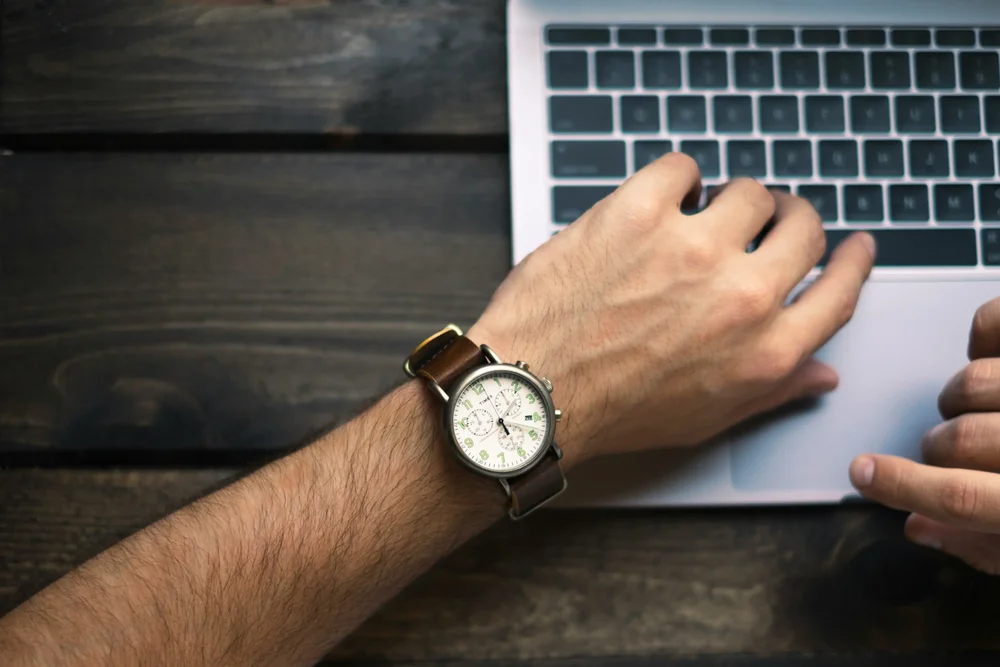
Speeded Test vs Power Test
The concept of Speeded vs Power Test is one of the ways of differentiating psychometric or educational assessments. In the context of educational measurement and depending on the assessment goals and time constraints, tests are
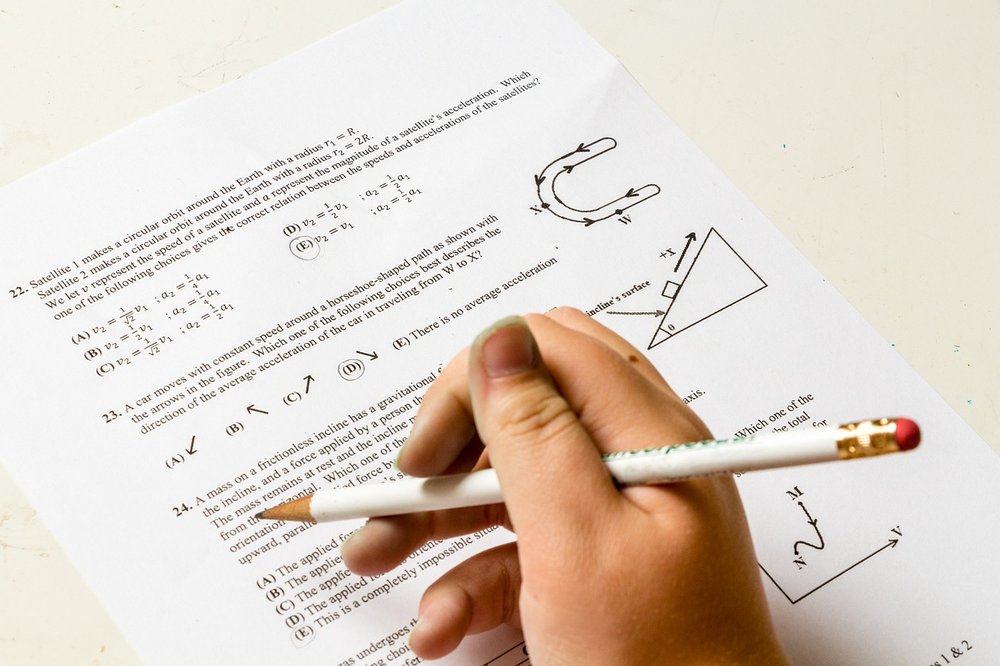
Educational Assessment of Mathematics
Educational assessment of Mathematics achievement is a critical aspect of most educational ministries and programs across the world. One might say that all subjects at school are equally important and that would be relatively true.
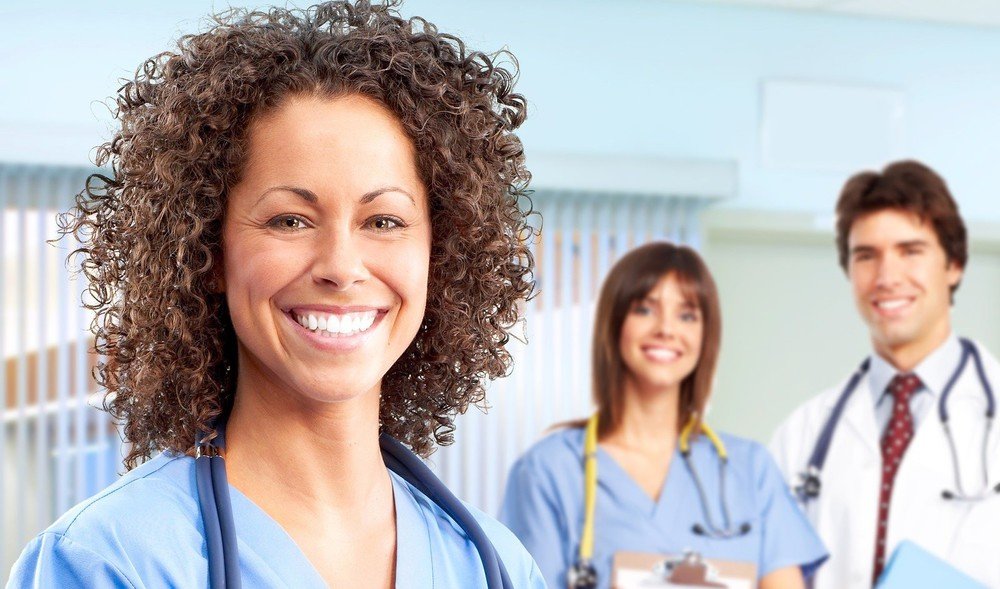
Objective Structured Clinical Examination (OSCE)
An Objective Structured Clinical Examination (OSCE Exam) is an assessment designed to measure performance of tasks, typically medical, in a high-fidelity way. It is more a test of skill than knowledge. For example, I used to
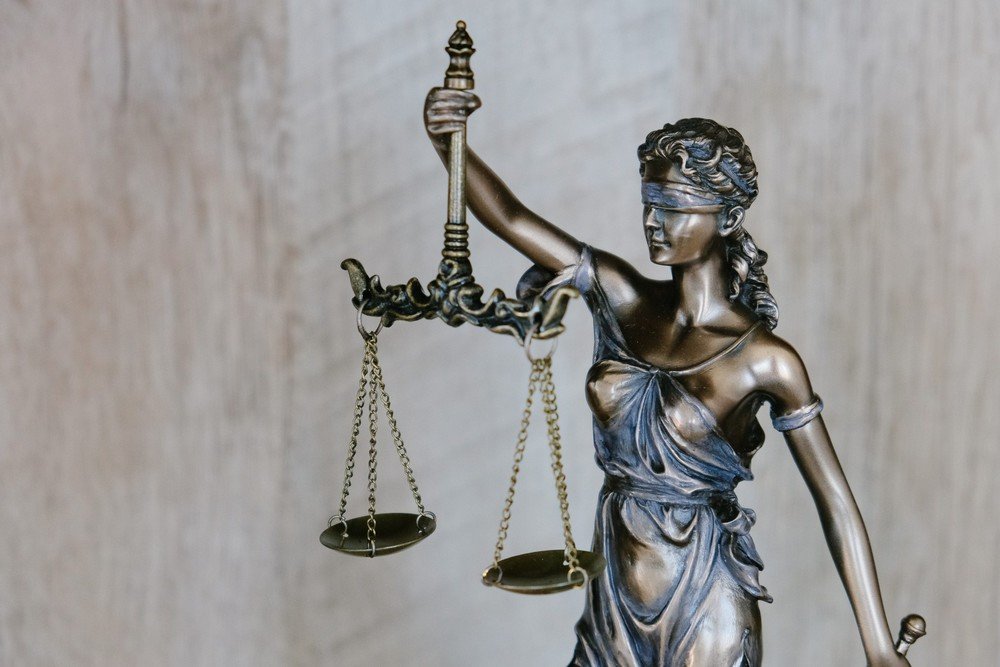
What Makes a Test Biased or Unfair?
One of the primary goals of psychometrics and assessment research is to ensure that tests, their scores, and interpretations of the scores, are reliable, valid, and fair. The concepts of reliability and validity are discussed
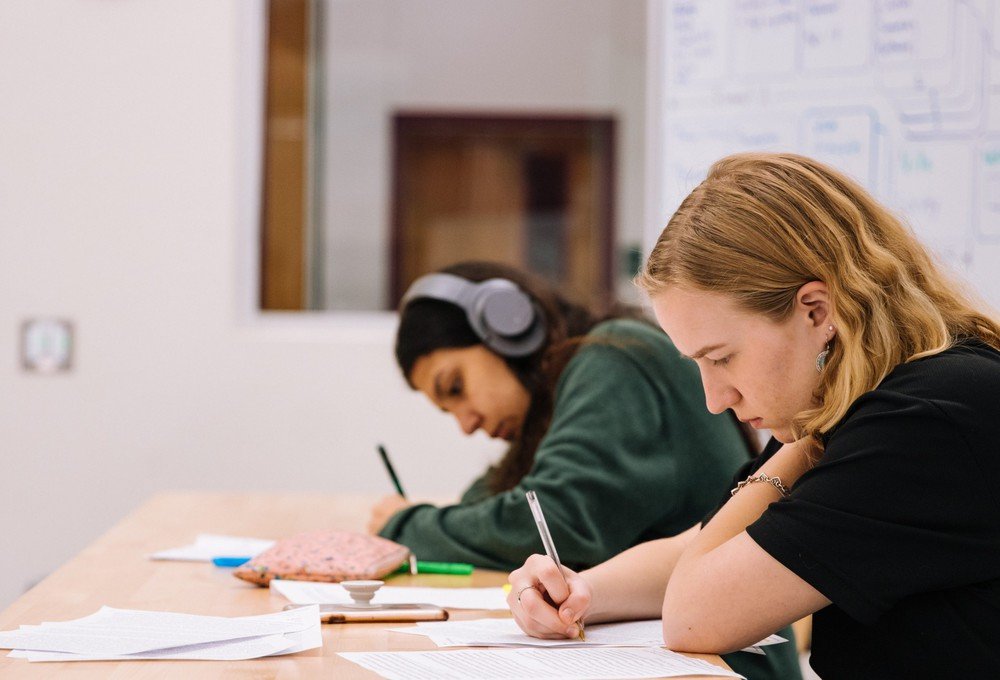
Progress Monitoring in Education
Progress monitoring is an essential component of a modern educational system and is often facilitated through Learning Management Systems (LMS), which streamline the tracking of learners’ academic achievements over time. Are you interested in tracking
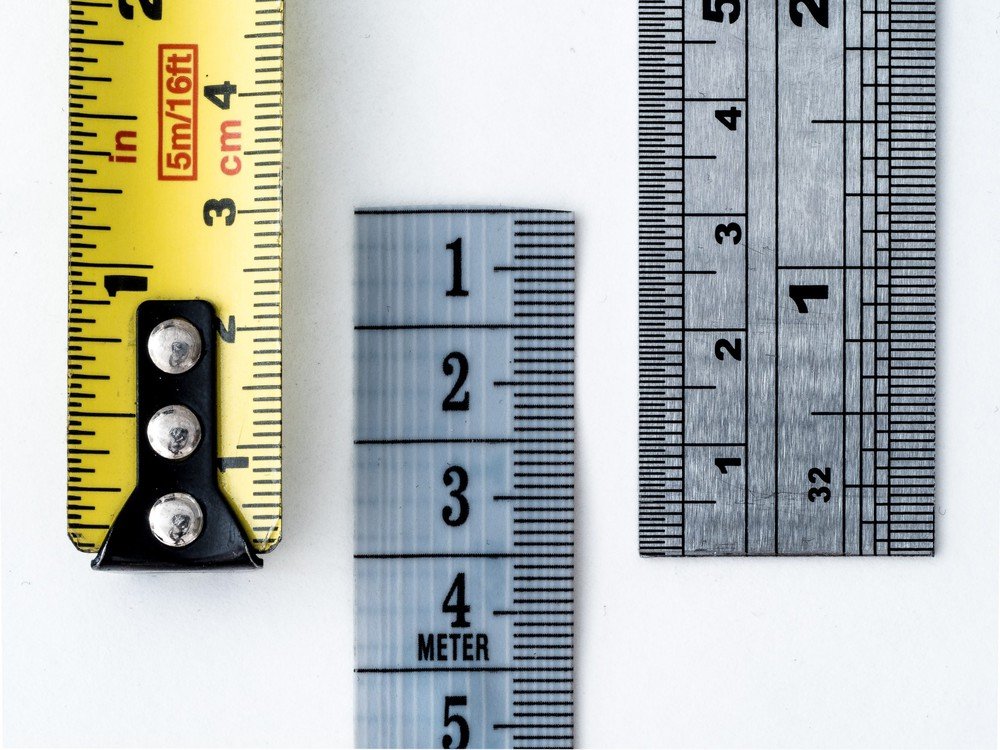
What is Vertical Scaling in Assessment?
Vertical scaling in educational assessments is the process of placing scores that measure the same knowledge domain but at different ability levels onto a common scale (Tong & Kolen, 2008). The most common example is
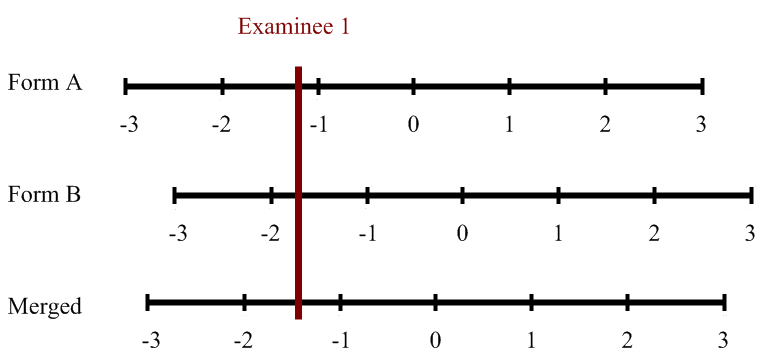
Test Score Equating and Linking
Test equating refers to the issue of defensibly translating scores from one test form to another. That is, if you have an exam where half of students see one set of items while the other
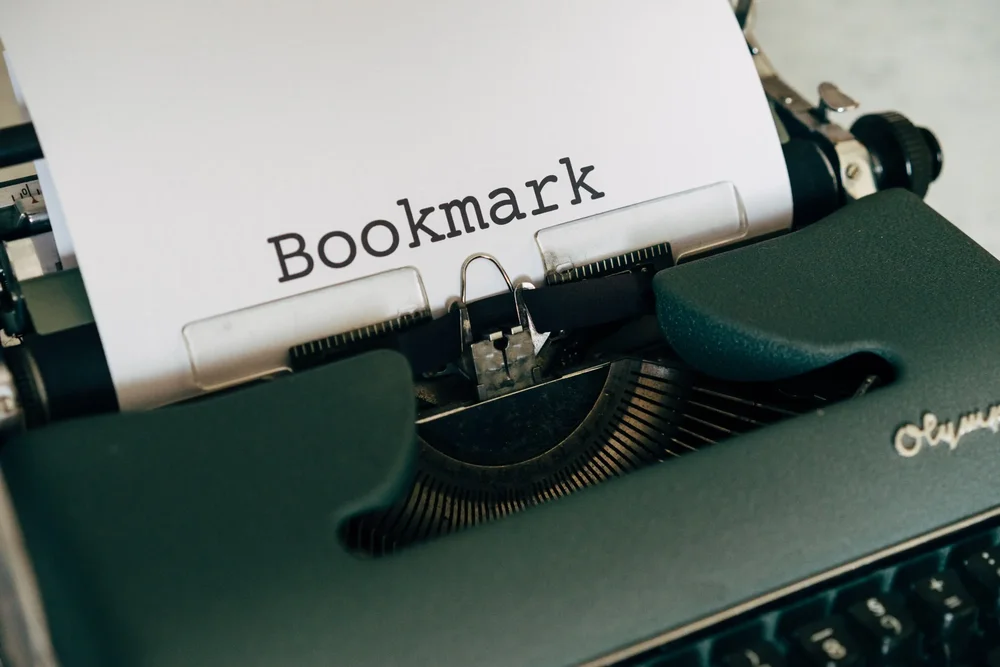
The Bookmark Method of Standard Setting
The Bookmark Method of standard setting (Lewis, Mitzel, & Green, 1996) is a scientifically-based approach to setting cutscores on an examination. It allows stakeholders of an assessment to make decisions and classifications about examinees that
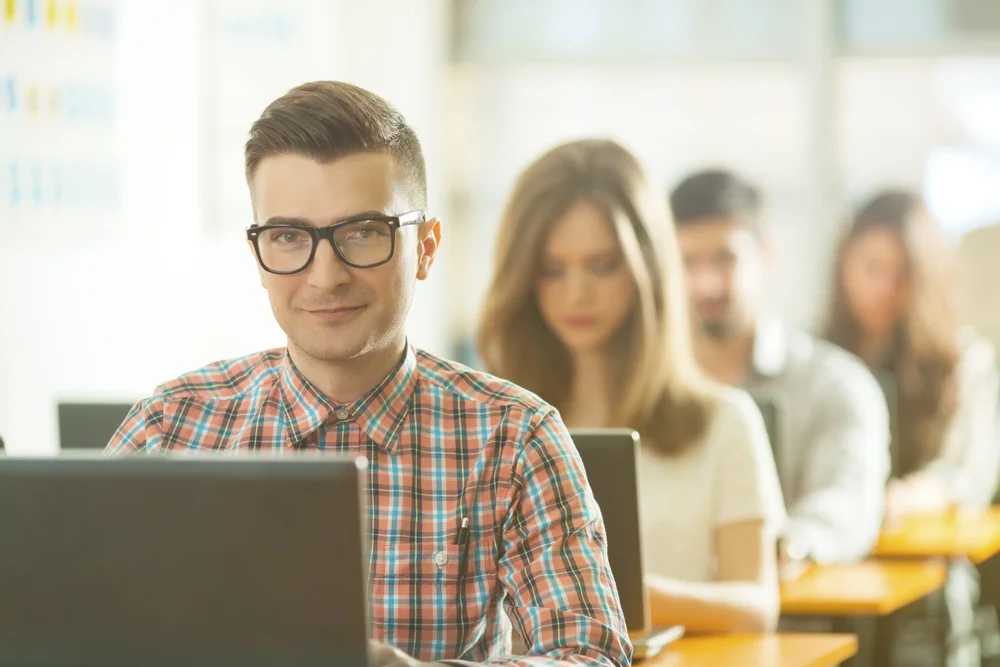
What is an Assessment / Test Battery?
A test battery or assessment battery is a set multiple psychometrically-distinct exams delivered in one administration. In some cases, these are various tests that are cobbled together for related purposes, such as a psychologist testing
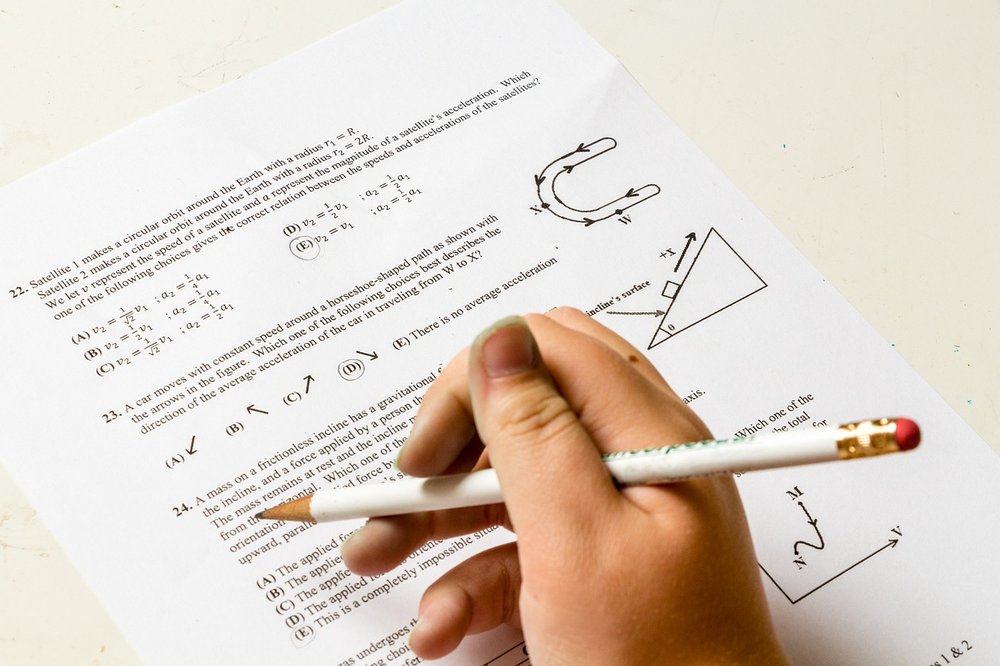
Paper-and-Pencil Testing: Still Around?
Paper-and-pencil testing used to be the only way to deliver assessments at scale. The introduction of computer-based testing (CBT) in the 1980s was a revelation – higher fidelity item types, immediate scoring & feedback, and
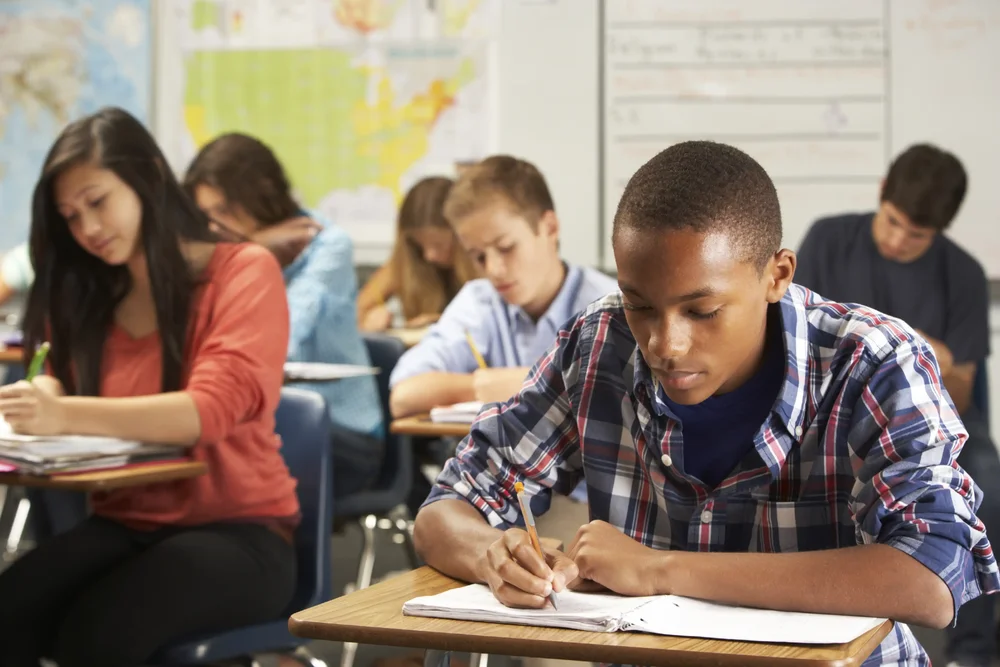
Tips to Select a Student Assessment Tool
With the overcrowded digital assessment marketplace, it can be hard to decide which online assessment tool will work for you.
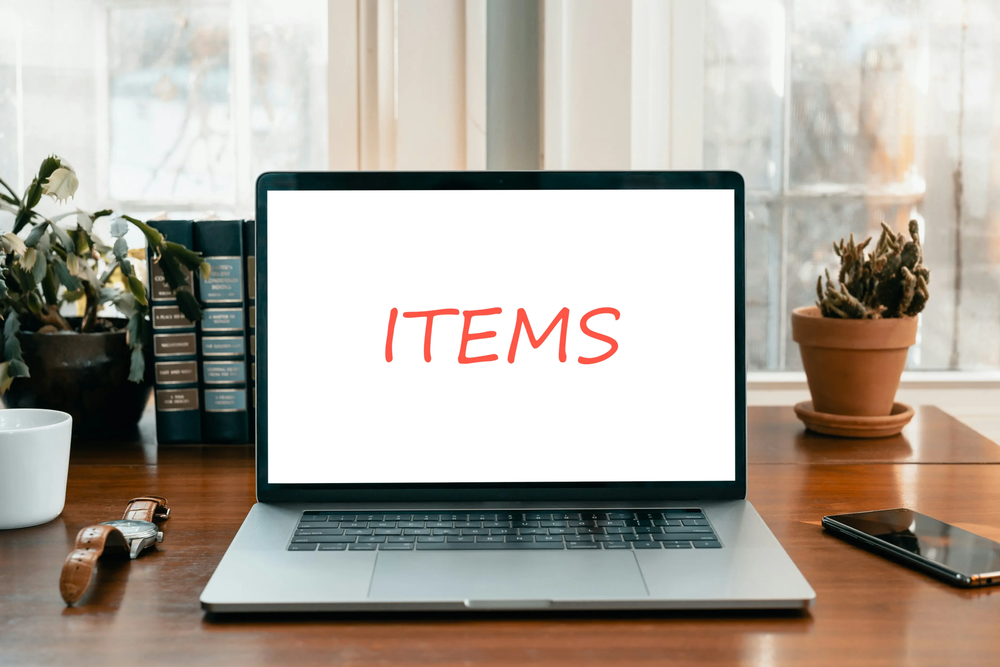
What is Item Banking? What are Item Banks?
Item banking refers to the purposeful creation of a database of assessment items to serve as a central repository of all test content, improving efficiency and quality. The term item refers to what many call
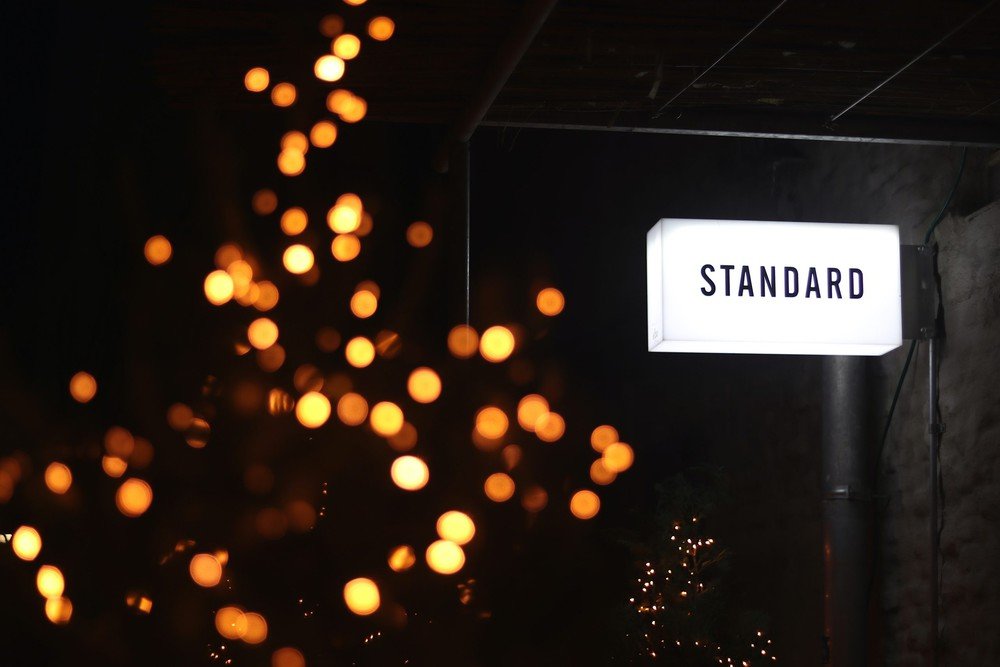
Ways the Word “Standard” is used in Assessment
If you have worked in the field of assessment and psychometrics, you have undoubtedly encountered the word “standard.” While a relatively simple word, it has the potential to be confusing because it is used in
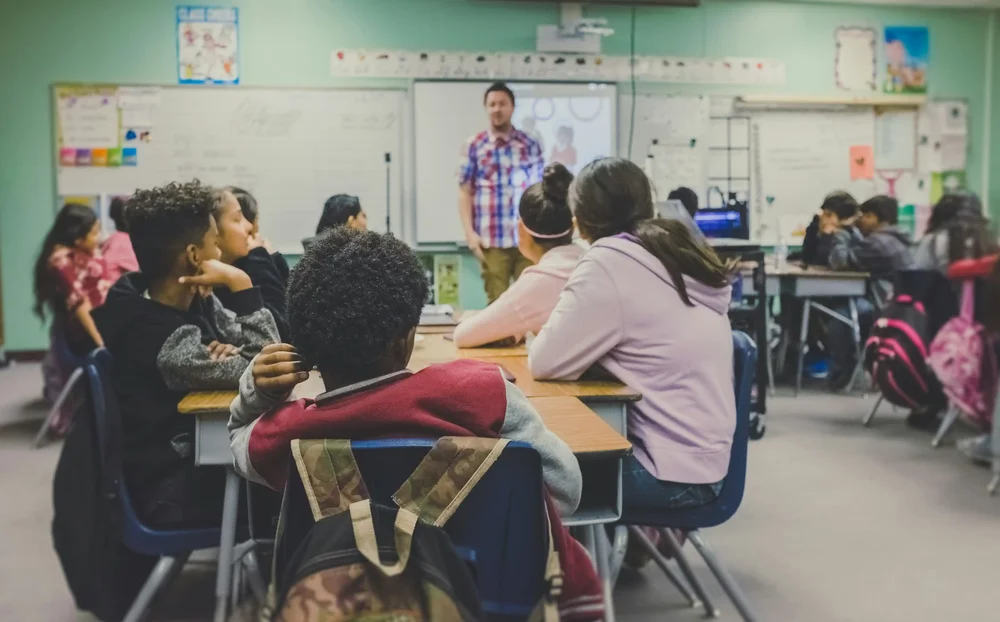
Is teaching to the test a bad thing?
One of the most cliche phrases associated with assessment is “teaching to the test.” I’ve always hated this phrase, because it is only used in a derogatory matter, almost always by people who do not
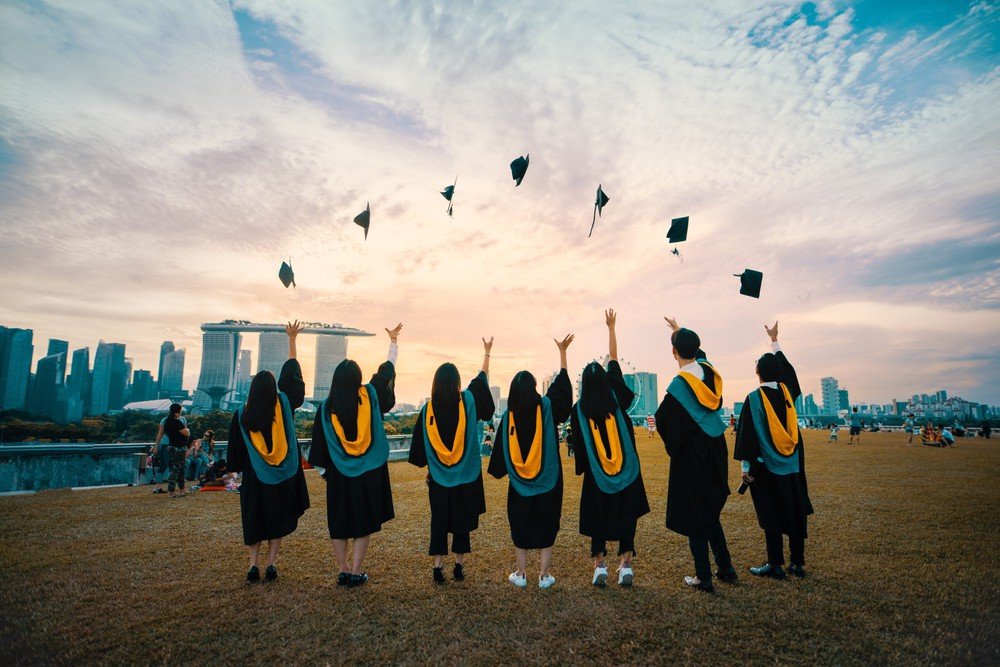
How to get a graduate degree or training in psychometrics
I’m also frequently asked by people already in the field where they can go to get a graduate degree in psychometrics, especially on sophisticated topics like item response theory...

What is the Sympson-Hetter Item Exposure Control?
Sympson-Hetter is a method of item exposure control within the algorithm of Computerized adaptive testing (CAT). It prevents the algorithm from over-using the best items in the pool. CAT is a powerful paradigm for delivering tests